Bibliography
Brown, A. (2018, 10 24). www.techopedia.com. Retrieved from Data Catalogs and the Maturation of the Machine Learning Market: https://www.techopedia.com/data-catalogs-and-the-maturation-of-the-machine-learning-market/2/33425
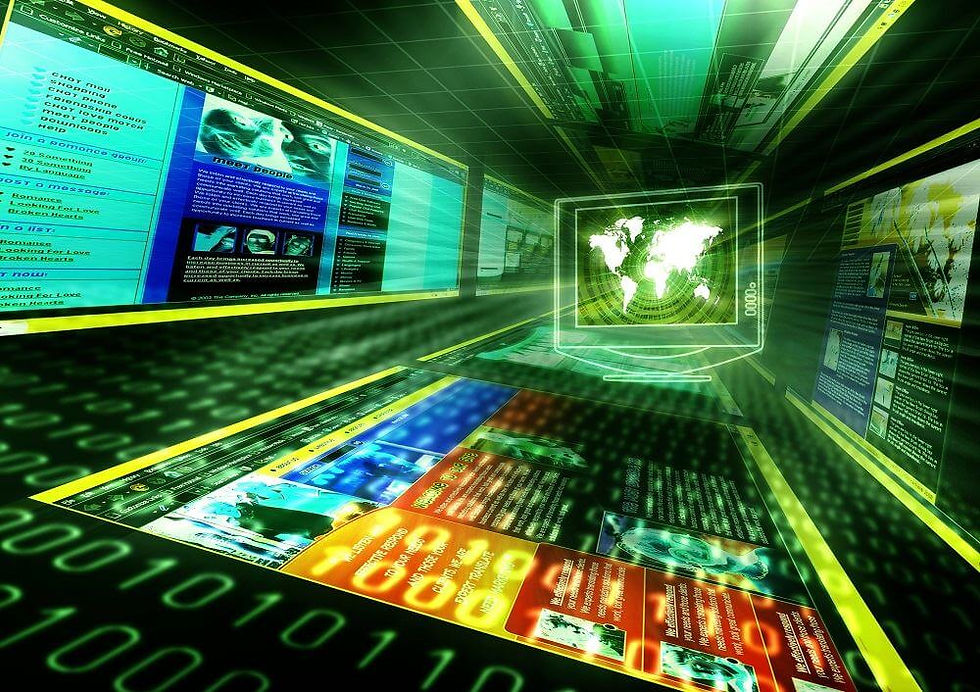
Takeaway: The MLDC market is growing, and enterprises seeking to effectively leverage big data with machine learning should be aware of the top names in the field and their individual rankings.
his is the age of big data. We get inundated with information, and businesses find it a challenge to manage and extract the value from it. Today's flow of big data entails not just volume, variety and velocity, but also complexity. As identified by SAS in Big Data History and Current Considerations that's a factor of the streams "from multiple sources, which makes it difficult to link, match, cleanse and transform data across systems." (Want to learn more about big data? Check out (Big) Data's Big Future.)
Finding valuable insight is not a question of simply amassing as much data as possible, but of finding the right data. It's impossible to work through it all with manual processes. This is why more and more businesses are "turning to data catalogs to democratize access to data, enable tribal data knowledge to curate information, apply data policies, and activate all data for business value quickly."
This is where data catalogs (sometimes also known as information catalogs) enter in the picture. As defined here, they empower "users to explore their required data sources and understand the data sources explored, and at the same time assist organizations to achieve more value from their present investments." One of the ways it does that is by enabling much greater access to data, among different types of users that can make use of or contribute to it.
The Infonomics Imperative
Noting the dramatically increased demand for data catalogs at the end of 2017, Gartner dubbed them "the new black." They were becoming recognized as a quick and economical solution "to inventory and classify the organization's increasingly distributed and disorganized data assets and map their information supply chains." The necessity for this has arisen due to the rise of "infonomics," which calls for applying the same meticulousness to tracking information as one does to managing other business assets. (For more on supply chains, see How Machine Learning Can Improve Supply Chain Efficiency.)
Gartner's take jibes with The Forrester Wave™: Machine Learning Data Catalogs, Q2 2018. Over half of the survey participants in that report said they were planning on building up their data catalog implementation. Likely they were largely motivated by the fact that each had at least seven data lakes in their organization. As the Gartner take on data catalogs explains, data catalogs are particularly useful for pulling out "the context, meaning and value of data" that is typically left in an unclassified form in a data lake.
Forrester reports that more than a third of data and analytics decision-makers were dealing with 1,000TB or more data in 2017, an amount reported by only between 10 and 14 percent the year before. Managing data on that scale is a growing challenge, or specifically, two challenges:
“1) merging existing business processes to source data to analyze it and implement insights and 2) sourcing, gathering, managing, and governing the data as it grows.”
What Data Catalogs Can Do for Businesses
Gartner identifies specific ways in which data catalogs can improve an organization's flow of information and productivity:
Collating and communicating the up-to-date information asset inventory that is available to the organization.
Creating the common glossary of business terms that defines the semanticinterpretation and meaning of the organization's data, thereby providing the means for mediating and resolving definitional inconsistencies.
Enabling a dynamic and agile collaboration environment to enable business and IT colleagues to comment on, document and share data.
Providing data usage transparency with lineage and impact analysis.
Monitoring, auditing and tracing data in support of information governance processes.
Capturing metadata to enhance internal analysis of data use and reuse, query optimization and data certification.
Contextualizing information within its business usage by capturing, communicating and analyzing what data exists, where it comes from, what contexts it is used in, why it is needed, how it flows between processes and systems, who is accountable for it, what it means and what value it has.
Getting the data properly identified and accessible to the key people in the organization is important, the Gartner report says, not just for finding the way "to monetize data assets for digital business outcomes," but to comply with regulations, whether they are industry-specific like the Health Insurance Portability and Accountability Act (HIPAA) or of a more general nature like the General Data Protection Regulation (GDPR).
Adding In Machine Learning
But nothing is without its drawbacks. For data catalogs, the problem has been the slow and tedious process entailed in manually building them up with all the metadata that needs to be put into place. This is where the machine learning component comes in.
The data catalogs that Forrester assessed are called MLDCs because they harness the power of machine learning, one of the components of AI. As a Podium Data blogexplained, that makes it possible to "build a persistent repository of metadata and then apply ML/AI to ferret out and expose potentially useful insights around underlying data assets."
How to Choose
To help organizations assess which one businesses should select, Forrester applied 29 points of evaluation to the top 12 MLDCs. It identified the leaders in this market as: IBM, Relito, Unifi Software, Alation and Collibra. The strong performers it found are Informatica, Oracle, Waterline Data, Infogix, Cambridge Semantics and Cloudera. Hortonworks stands alone in the rank of "contender."
However, one should not go by the overall rankings alone. The report does break down the particular strengths and weaknesses of each one. Accordingly, if a particular feature, like research and development, is of the utmost importance for an organization, it may consider Hortonworks as the equal of IBM and Colilbra for that aspect because those three share the top score of five for that quality, which was two points better than Alation and Coloudera and four points better than Cambridge Semantics.
Accordingly, the Forrester report advises those who use its report for guidance to not assume the top ranked company is the best choice for everyone. They should pay close attention to the breakdown of the assessment to find what meets their particular requirements.