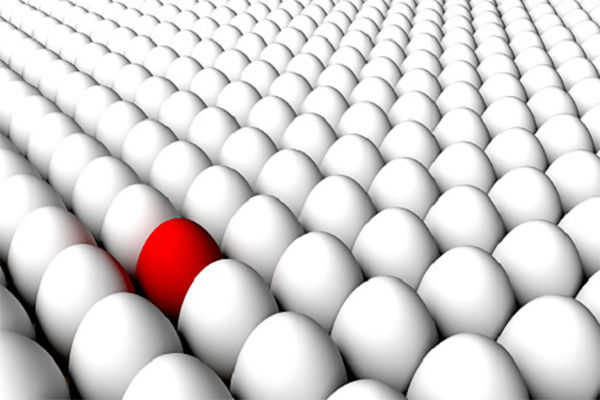
Finding the exception is the role of anomaly detection software, which is made more effective with AI. A new model called MIDAS is said to be faster and more accurate.
Anomaly detection is work to identify rare events or observations that differ in a big way from the majority of surrounding data, thus raising questions as to why it is the case.
Anomaly detection, synonymous with outlier detection, is used in many fields including statistics, finance, manufacturing, networking and data mining. It can be useful for intrusion detection, fraud detection, system health monitoring and event detection in sensor networks. It is used in preprocessing to remove irregular data from the dataset, which can substantially increase accuracy.
Today anomaly detection is also used in cyber security for spam filters, credit card fraud detection, network security and social media content moderation.
A new approach to anomaly detection from researchers at the National University of Singapore is said to outperform baseline approaches in speeds and accuracy, according to a recent account in KDnuggets. Called MIDAS, for Microcluster-Based Detector of Anomalies in Edge Streams, the system was developed by PhD candidate Siddharth Bhatia and his team.
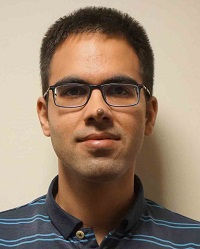
Siddharth Bhatia, PhD student, National University of Singapore
MIDAS is able to detect irregular data in microclusters, which are fine particles with properties that can be measured. The irregularities or anomalies in the data can be detected in realtime at speeds said to be many times greater than existing, state-of-the-art models.
“Anomaly detection in graphs is a critical problem for finding suspicious behavior in countless systems,” stated Bhatia. “Some of these systems include intrusion detection, fake ratings, and financial fraud.”
Social networks such as Twitter and Facebook could use the technology to help detect fake profiles used for phishing and spam. “Using MIDAS, we can find anomalous edges and nodes in a dynamic (time-evolving) graph,” stated Bhatia. “In Twitter and Facebook, tweet and message networks can be considered a time-evolving graph. We can find the malicious messages and fake profiles by finding the anomalous edges and nodes in these graphs.”
To research the potential of MIDAS in social network security and intrusion detection tasks, Siddharth and his team used the following datasets for anomaly detection: Darpa Intrusion Detection (4.5 million IP-IP communications); Twitter Security Dataset (2.6 million tweets related to security events in 2014); and the Twitter World Cup Dataset (1.7 million tweets during World Cup Soccer in 2014).
The results showed microcluster anomalies could be detected using MIDAS with 48 percent more accuracy and 644 times faster than baseline approaches. “We think it will become a new baseline approach and be quite useful for Anomaly Detection,” stated Bhatia. “Also, it will be interesting to explore how MIDAS can contribute in other applications.”
Asked by AI Trends how MIDAS makes use of AI, Bhatia responded, “MIDAS uses unsupervised learning to detect anomalies in a streaming manner in real-time. It was designed keeping in mind the way recent sophisticated attacks occur. MIDAS can be used to detect intrusions, Denial of Service (DoS), Distributed Denial of Service (DDoS) attacks, financial fraud and fake ratings. MIDAS combines a chi-squared goodness-of-fit test with the Count-Min-Sketch (CMS) streaming data structures to get an anomaly score for each edge. It then incorporates temporal and spatial relations to achieve better performance. MIDAS provides theoretical guarantees on the false positives and is three orders of magnitude faster than existing state of the art solutions.”
Read the full paper from Siddharth Bhatia and colleagues. Bhatia is interning with Amazon AI Labs during the summer.
AI and Anomaly Detection
Modern anomaly detection relies heavily on AI to do its work. Statistical Process Control, or SPC, introduced in 1924, is the gold-standard methodology for measuring and controlling quality in the course of manufacturing. Fusing SPC with AI makes the technique more accurate and precise, according to a report from Sciforce, an IT consulting company based in Ukraine, published in Medium.
The ability of AI and machine learning-based systems to learn as they go and deliver more precision with each iteration, makes anomaly detection more effective. The stages are: feed datasets into the AI system; develop data models based on the datasets; see a potential anomaly each time a transaction deviates from the model; have a domain expert approved the deviation as an anomaly; the system learns from the action and builds on the data model for future predictions.
The consultants used supervised machine learning models to label a training set with normal and anomalous samples for constructing a prediction model. The most common supervised methods include supervised neural networks, support vector machines, k-nearest neighbors, Bayesian networks and decision trees.
The consultants were also familiar with unsupervised techniques, which do not require manually labeled training data. They presume most of the network connections are normal traffic and assume only a small amount is abnormal. The most popular unsupervised models are K-means, Autoencoders, GMMS and hypothesis tests-based analysis.
“Like probably any company specialized in Artificial Intelligence and dealing with solutions for IoT, we found ourselves hunting for anomalies for our client from the manufacturing industry,” the Sciforce team reported. “Using generative models for likelihood estimation, we detected the algorithm defects, speeding up regular processing algorithms, increasing the system stability, and creating a customized processing routine which takes care of anomalies.”
Commercial use requires more work. For that, “Anomaly detection needs to encompass two parts: anomaly detection itself and prediction of future anomalies.”
The team concluded that anomaly detection alone, or coupled with the prediction functionality, can be an effective means to catch fraud and discover strange activity in large and complex datasets. This can be crucial for security in banking, medicine, manufacturing, natural sciences and marketing, which depend on smooth operations. “With Artificial Intelligence, businesses can increase effectiveness and safety of their digital operations,” the authors state.