Executive Interview: Dr. David Bray, Director, Atlantic Council
- COVID-19 Pandemic Has Accelerated the Rate of
- Jul 30, 2020
- 9 min read
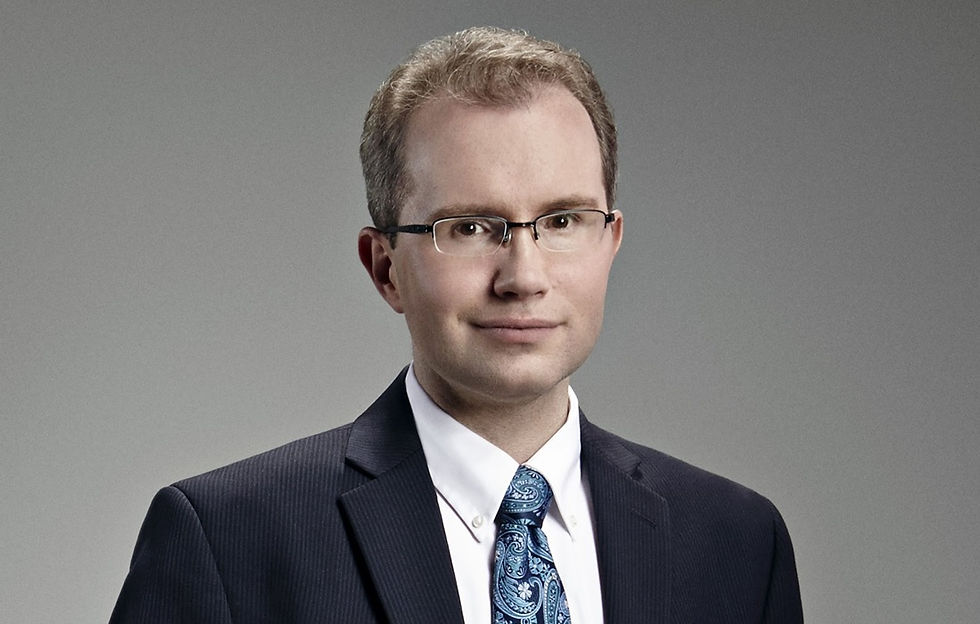
Dr. David Bray is the Inaugural Director of the new global GeoTech Center & Commission of the Atlantic Council, a nonprofit for international political, business, and intellectual leaders founded in 1961. Headquartered in Washington, DC, the Council offers programs related to international security and global economic prosperity. In previous leadership roles, Bray led the technology aspects of the Centers for Disease Control’s bioterrorism preparedness program in response to 9/11, the outbreak response to the West Nile virus, SARS, monkey pox and other emergencies.
He also spent time on the ground in Afghanistan in 2009 as a senior advisor to both military and humanitarian assistance efforts, serving as the non-partisan Executive Director for a bipartisan National Commission on R&D, and providing leadership as a non-partisan federal agency Senior Executive focused on digital modernization. He also is a Young Global Leader for 2017-2021 of the World Economic Forum.
David Bray, Director, GeoTech Center & Commission, Atlantic Council
Bray is a member of multiple Boards of Directors and has worked with the U.S. Special Operations Command on counter-misinformation efforts. He was invited to give the 2019 UN Charter Keynote on the future of AI & IoT governance. His academic background includes a PhD from Emory University; he also has held affiliations with MIT, Harvard, and the University of Oxford. He recently took a few moments to speak to AI Trends Editor John P. Desmond about current events, including the geopolitics of the COVID-19 pandemic.
AI Trends: Thank you David for talking to AI Trends today. We will start with the Coronavirus since it’s so topical today, then expand out. What role do you see AI playing in the fight against COVID-19?
Dr. David Bray: With AI, we are dealing with something that really is historically unprecedented. The last pandemic that we had was the Spanish influenza [of 1918-1919] and that was, obviously, before all the advances in computers and data and AI.
So, what we need to do first and foremost is assemble good data on what we are seeing, because especially with machine learning models and trying to train the machine, this really is unprecedented territory. We currently lack sufficient data sets to train on that can help predict the future given the unprecedented nature of this pandemic. What we are discovering is that when it comes to bringing together data about COVID-19 and the pandemic, different countries, different regions, even different sectors are either unintentionally, or in some cases intentionally, biasing their data sets. That may just be because they are waiting on when they report the numbers, or what they consider a case versus not a case of COVID-19.
This absence of good quality data is making it really hard right now to use any type of machine learning or algorithms to inform both the immediate response, and even more importantly, how do we rebuild? Yet this absence of good quality data is an area where statistical techniques and some AI techniques might be able to help to at least begin to identify and curate better data sets. For instance, are we seeing anomalies in the data that look like spikes, but in fact may be because some regions might report every seven days? For COVID-19, before we can use AI, the first step is getting good quality data.
We are also seeing where AI is playing a role in trying to make sense of all the scientific literature about the virus. I have seen statistics that say we need to wade through 25,000 different articles, not all of them peer-reviewed yet, on what we know about the virus. I’ve seen others that say it’s upwards of 50,000 or more; either way, the number of articles are way more than any one human or even a team of humans can wade through. AI approaches can help us inform a novel therapy or a novel intervention that might help us address what is going on with the pandemic.
How should an AI-related global effort to fight the coronavirus be taking shape? How do you envision it?
We are seeing a fragmentation of systems in place that we had a high degree of confidence would have been able to respond to this. That is partly because a lot of these institutions involving how nations relate to each other and how nations coordinate, were put in place after World War II.
After all, back in the 1970s we eradicated smallpox. Yet now in 2020, we are discovering that, unfortunately, funds for states in the United States to have available capacity to characterize and respond to outbreaks appear to not have been sufficient. Leaders may have convinced themselves with past responses to outbreaks of Ebola, HIN1 influenza and other things, that we could handle this and subsequent public health budgets focused on pandemic preparedness waned, resulting in a loss of expertise, equipment, and experience in responding to outbreaks.
Beyond internal coordination challenges in the United States, the COVID-19 pandemic is shining a light on the reality that ways of coordinating globally have broken down. We are seeing every nation sort of turn inwards and even within the nations, fragment regionally.
COVID-19 also may be illuminating that the world may be in this era in which things that used to be done by government cannot solely be done by government anymore. There are global activities that used to be done solely by government that now need to be done by industry as a partner. Yet industry does not really know how to make a case beyond increasing ROI, increasing profits, and increasing shareholder value. So for open societies that do distinguish between the work of their public vs. private sector, what we need to do is find some way to bring together both industry and governance mechanisms that involve the public to respond to address global challenges such as the COVID-19 pandemic.
I will put these concerns in a pragmatic context with regards to data and AI. In some countries responding to COVID-19, all the data about their people belongs to the nation-state. I’m glad that, in the United States, we don’t take that approach and that we are not a surveillance state. Yet at the same time the different levels of government and differences between data that the public vs. private sector has fragments the ability of the United States and other open societies. We must find better ways to bring good data forward, with consent and via a decentralized method that is not surveillance state, to inform the COVID-19 response.
In the United Kingdom, back in 2017, the government identified a potential solution called Data Trusts. The United Kingdom proposed it to overcome the challenge that the UK does not have as much data as China does to train machines. Data Trusts would involve transparency, auditability, and a framework to involve individuals and companies for a time-defined, focused effort to share data for a specific purpose.
With Data Trusts, it is clear why the data is being brought together; it was not to make any profits or to inform the surveillance state, but for a specific purpose. In this case, a Data Trust could be informing on what we should do with COVID-19.
Unfortunately, while the UK government was starting to consider doing pilots on this in 2020 before the virus hit. With the Atlantic Council GeoTech Center, we have been emphasizing Data Trusts are needed to bring together data and inform the long-term COVID-19 recovery. Open societies need to work together to figure out a way to bring together data that is neither surveillance state or autocracy. There are several questions that need answers that require better quality data sets, such as is there going to be a second wave of COVID-19? How do we best pursue the long-term recovery needed for the world?
Some good thoughts there. Can I ask, the recent posting of the Atlantic Council suggests that the COVID-19 pandemic might lead to more adoption of AI: as complex supplier networks are restructured, as AI use by online retailers accelerates, and as spending is targeted to revive the manufacturing base and reskill employees. Could you expand on any of these?
We have been having conversations with experts as well as polling different experts in how technology and data intersect with geopolitics. What we’ve heard and what we found is that COVID-19 has accelerated trends that previously many people thought would take another 10 to 15 years. Before COVID-19, we were inching towards more digitization and modernization, remote work, and autonomous ways of manufacturing. COVID-19 has accelerated these trends. What would probably have taken 10 to 15 years will probably now be more like two to four years as the pandemic creates a digitization imperative.
For example, anyone who right now has a manufacturing factory that is heavily dependent on having people present at the factory, such factory owners or investors are probably looking at a future where they will at least pursue some semi-autonomous, if not full autonomous, manufacturing so the factory can continue to work if the humans aren’t there.
This pandemic’s acceleration on autonomous manufacturing is going to move us to more distributed ways of doing manufacturing that are not dependent on large global supply chains. We will see advances in additive manufacturing and in 3D printing. Moving to embrace distributed and autonomous ways of manufacturing will also raise questions of how do you do quality control? How do you make sure what is done in region A is as good as region B or C?
With the GeoTech Center, we are monitoring advances using computer vision and AI to watch each step of what a person does or what a machine does. If the machine in step three or four does something that’s slightly different or slightly that’s not in keeping with consistency, the machine can recommend a corrective step. Or if the manufacturing error was so egregious, the machine can label what was being produced as defective and put it aside.
As societies, post-COVID-19 we may shift to a more localized, distributed, resilient production enabled by AI, while having the same level that centralized quality assurance would provide or better. A company that’s leading in this space is Nanotronics. [Ed note: The company is a nanotechnology startup in Cuyahoga Falls, Ohio, with an office in Brooklyn, New York at New Lab, and manufacturing operations in California.]
The GeoTech Center also is monitoring the future of work and the future of education. COVID-19 has accelerated online learning in response to the new skills required for jobs of the future. Higher education is likely to have a strong online component going forward that may also be experiential-based or team-based with some in-person component as well. For the decade ahead, individuals will be comparing the value proposition of spending four years at an expensive location for college, versus interning in a work setting and gaining experiences while learning remotely.
In addition, for delivery of education, it is likely to be tailored to deliver materials online based on how a student learns. Data and AI can help identify if a student is more visual or prefer to hear things via podcasts.
Shifting to data, how much of a challenge is it to aggregate the disparate data needed to train AI systems?
For a COVID-19, because it is unprecedented, aggregating the disparate data needed to train AI systems is hard. I have talked to colleagues at different companies as well as different governmental organizations. Part of the challenge is sometimes the data does not even exist yet that is needed to train AI for the pandemic. Or if the data does exist, it has a fast rate of decay.
We are dealing with a very fast-moving, turbulent environment in which the data may not exist or it may decay quickly. There may be concerns about intellectual property or proprietary information. Some companies do not want to put their proprietary information at risk and some individuals do not want to put their personalized information at risk of misuse.
This is why I really think Data Trusts are interesting because, essentially, they can help bring together people towards a common purpose associated with data. Data Trusts should have transparency about the audit mechanisms to ensure the effort is protecting and treating the data appropriately. Data Trusts also help address proprietary information concerns, because while they can involve open data, they can also involve closed data such as personal data or proprietary data as well.
We have seen some early signs of good approaches to bringing together data across sectors and nations. Microsoft does have an open source data effort {Ed. Note: See The Economist.}, which is, I think a really great example. Since such an effort is open source, it won’t assemble personalized or proprietary information. If this open source data effort were to pursue a Data Trust approach with transparency, audit mechanisms, and bringing in people from whatever localities are being impacted by how this data is informing what we should do – it could be expanded to incorporate data sets of value to inform the long-term COVID-19 recovery.
I think it is important to involve members of the public as part of the oversight of any Data Trust. This is so the representatives can say, “I see you’re using that data for this purpose, but that’s not actually right or that’s not fully representative of us.” Maybe the data is skewed towards a certain demographic. The public can also encourage the Data Trusts to consider the time-horizon of such an effort. For example, after 30, 60 or 90 days, the data can be forgotten and the Data Trust can ensure its efforts are not used for other purposes beyond the transparent purpose of the effort.
What would be the best way to collect data about the COVID-19 virus? Where would the data come from, in your opinion ideally?
Ideally, data would come from people opting in and not having to do too much to provide the data. With the COVID-19 pandemic there has been a lot of effort towards contact tracing. We have seen Google and Apple launch contact tracing. I think that is well-intended. Based on my background with public health and bioterrorism preparedness, a concern I have is contact tracing really depends on good testing. We know that testing still remains a challenge here in the United States. Even when tests are available, we have some false positives and false negatives. When you get to larger than 50,000 people, and you have false positives and false negatives, the data analyses risk getting confused as a result.