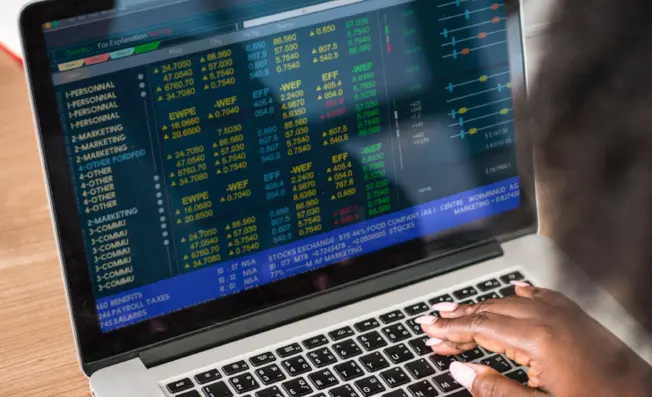
AI and machine learning have moved from the fringes to the very centre of the technology debate. But which industry has the huge datasets, along with the capital and staffing resources, to make the most of AI? As recently reported by both PwC and Gartner, it is the financial sector.
AI in the fintech industry
We at Netguru have picked out 5 areas where AI and ML are calling the shots in banking and fintech. Are you wondering how to use AI in fintech and what are the latest banking and fintech AI trends? Are you eager to learn more about problems of AI in fintech? Read on.
1. Automated Customer Support - Customer Service Redefined
Customer service is the most obvious place where improved AI already assists (and may eventually replace) humans. Chatbots and AI interfaces like Cleo, Eno, and the Wells Fargo Bot interact with customers and answer queries, offering massive potential to cut front office and helpline staffing costs. The London-based financial-sector research firm Autonomous produced a report which predicts that the finance sector can leverage AI technology to cut 22% of operating costs - totaling a staggering $1 trillion.
Founded in 2016, Cleo is an AI assistant that intelligently manages your money
In the back and middle office, AI can be applied in areas such as underwriting, data processing or anti-money laundering. But it is the front office use of chatbots that is the visible ‘face’ of the technology. Kai-Fu Lee, CEO of the investment firm Sinovation Ventures, which specializes in AI and high-tech ventures, predicts that bank teller is the first profession that will be replaced by AI. Although it will be at least a decade before the experience of communicating with an AI is seamless enough for it to totally replace human customer service.
Natural Language Processing (NLP) is really the key here - utilizing deep learning algorithms to understand language and generate responses in a more natural way. Swedbank, which has over a half of its customers already using digital banking, is using the Nina chatbot with NLP to try and fully resolve 2 million transactional calls to its contact center each year.
While customers seem to be aware of the prominence of chatbots, they are barely aware of the existence of the key importance of NLP in AI. Take a look at this graph, based on Edelman's report on the awareness of AI.
Although the awareness may be low, it is clear that there is no future customer service without AI involved. And banks have certainly got the message; with 95% stating that they plan to increase the use of chatbots in the coming years, with some estimating that chatbots will handle 80% of all customer interactions by 2020.
2. Client Risk Profile - Faster and More Reliable Credit Scores
There is a saying among corporate bankers “You only get credit if you don’t need it”. Translated into business terms: there were underserved customers, ignored by traditional financial industry, who couldn’t apply for a loan or credit. And that helped a lot of fintech startups, the likes of Finiata (Netguru is proud to be a partner here) or Lenddo, to put their foot in the door.
Applications like Lenddo are bridging the gap for those who want to apply for a loan in the developing world, but have no credit history for the bank to review. Potential borrowers sign up and allow the app to mine their data from social media, web browser history, geo-locations, and other information, to build a picture that allows banks to assess if they are creditworthy or not--without any of the traditional metrics that make up the credit rating. And there is clearly appetite among the estimated 600 million potential lenders in developing countries who banks will not consider, as the startup managed to attract 350,000 users in just 2 years from launch.
In the developed world, social media (SoMe) data is used by microloan companies like Affirm in what they term a ‘soft’ credit score. They don’t need to compile a full credit history to lend small amounts for online purchasing, but SoMe data can be used to verify the borrower and do some basic background research. This is similar to some car loan companies, like Neo, which use LinkedIn profiles to verify that a person’s stated work history is genuine, cross-checking listed jobs against a user’s contacts on the site.
If these early attempts at using SoMe data for banking prove successful, it seems inevitable that companies like Amazon, Google and Facebook will start partnering with financial sector companies to provide them with profiling data.
In March 2019, Affirm had signed up a partnership agreement with Walmart to provide its services (and credit options) at the point-of-sales at the biggest retail chain in US.
3. Trading and Money Management
It seems like an anomaly, but humans are more likely to accept financial guidance from another human than from a smart machine. Incredibly, 46% of people would be willing to undergo AI-assisted surgery but only 34% would be comfortable with machine-led financial guidance.
Yet the industry is willing to alter these numbers. For instance, Citi Private Bank has been using machine learning to share - anonymously - portfolios of other investors to help its users determine the best investing strategies.
For many years it seemed that machine-led deep market analysis and prediction was so near and yet so far. Today, as business writer Bryan Borzykowski suggests, technology has caught up and we have both the computational power and the right applications for computers to beat human predictions.
One of the hottest trends in AI research are Generative Adversarial Networks (GANs). GANs are perceived as a big future technology in trading, as well as having uses in asset and derivative pricing or risk factor modelling. Using new models to make rapid advances in the way AI deals with unstructured data, GANs are able to solve a lot of the problems that AI has so far struggled to deal with using the limited and noisy data created in the banking industry.
Generative Adversarial Networks might change asset or strategic trading for good
The simple way to understand its relevance in the banking industry is to take this quote from Aldous Birchall, Head of Financial Services AI at PwC: “I would go so far as to say that any asset manager or bank that engages in strategic trading will be seriously competitively compromised within the next five years if they do not learn how to use this technology.”
4. Regulatory compliance
The advancement of AI and ML technology in the financial branch means that investment firms are turning on machines and turning off human analysts. Bryan Borzynski writes: “Bridgewater Associates, the world's largest hedge fund, said that it will replace many of its managers with machines. Research firm Optimas estimates that by 2025, AI use will cause a 10 per cent reduction in the financial services workforce, with 40 per cent of those layoffs in money management operations.”
But there are increasing calls, for example from established industry players like Derek Dempsey of FICO, for “Explainable AI” - a way to enhance accountability in areas such as investment and credit scoring. Artificial Intelligence can be used to calculate and analyse cash flows and predict future scenarios, for example, but it does not explain the logic or processes it used to reach a conclusion.
One of the brands that want to jump on the new trend is a company called Digite, IT provider of a tool RISHI-XAI. According to the Digite's founders, the tool can improve efficiency and profitability of any IT service project, by minimising delays.
Explainable AI is a response to this specific problem: calling for machines to explain their reasoning, particularly in areas like loan refusals, where their decisions directly affect humans. And in the GDPR-era this is a major issue. Banks want to leverage the technology, but they need to do so in an accountable way.
5. Security and Fraud-busting
The banking industry is probably the most obvious target for online fraud and hackers. Some of the most innovative AI and Machine Learning solutions are created in this area.
Netguru spoke to Nick Lally of Ravelin, a firm set up in 2014 to tackle fraudulent payments in the global on-demand economy. The application evolved from a taxi-hailing app when the team realized that account history data could be used to build a pattern of normal usage and identify payments that fall outside of that picture and may be fraudulent.
The big trend here is that the two-click purchases offer great convenience for shoppers, but also present huge opportunities for fraudsters. The financial gain for companies in stopping such activities is massive: a 2017 report by LexisNexis suggests that every dollar of fraud, a companies have to spend $3.37 to resolve the problem and appease the customer.
Also, Onfido, a company that helps businesses manage risk and prevent fraud during the user onboarding with the identify verification, published a series of white papers on how to leverage AI tools to defeat fraudulent transactions.
As the amount of data increases and machine learning helps identify weaknesses and solutions, it becomes essential to the innovation of new security solutions. There are any number of machine learning security enhancements that are already part of our everyday experience. In fact, Jack Gold, founder of J. Gold Associates states that, “Most of the major companies in security have already moved to a machine learning system that tries to interpret actions and events and learns from a variety of sources what is safe and what is not.”
As outlined in Netguru’s blog by Katarzyna Zachariasz, the key elements for good machine learning are enough time and human skill to develop the right algorithms, and vast resources of data that become fuel for the engine. In banking, every single transaction creates a wealth of data that can be analysed and optimised for usage.
The sector also has the money to employ the right people and give them the time to create killer applications. Therefore, if we are looking for great machine learning and AI innovations in the future, it makes sense to keep our eye on banking and fintech.
Watch This : https://www.youtube.com/watch?v=Z5vxRC8dMvs