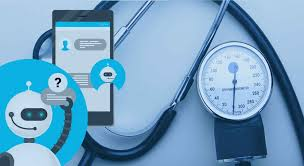
Medical diagnostics are a category of medical tests designed to detect infections, conditions and diseases. These medical diagnostics fall under the category of in vitro medical diagnostics (IVD) which be purchased by consumers or used in laboratory settings. Biological samples are isolated from the human body such as blood or tissue to provide results. Today, AI is playing an integral role in the evolution of the field of medical diagnostics.
We set out in this article to explore current applications of AI in medical diagnostics to help business leaders understand the trends that are shaping the field. We will present our findings in three main sections:
Background definitions and summarized insights from our research
Current applications of AI in medical diagnostics
Related Emerj executive interviews
Our own recent healthcare AI industry research suggests that around a third of all healthcare AI SaaS companies are focusing partly or exclusively on diagnostics – making it one of the largest focus areas for burgeoning startups in the field. Further detail on mobile medical diagnostics can be found within our article, AI for Mobile Medical Diagnostics – Current Applications.
Before diving into present applications, we’ll begin with background facts and terminology about in-vitro medical diagnostics, and a brief summary of the findings of our research on this topic:
Machine Learning for Medical Diagnostics: Insights Up Front
The Institute of Medicine at the National Academies of Science, Engineering and Medicine reports that “diagnostic errors contribute to approximately 10 percent of patient deaths,” and also account for 6 to 17 percent of hospital complications. It is important to note that physician performance is typically not the direct cause of diagnostic errors. In fact, researchers attribute the cause of diagnostics errors to a variety of factors including:
Inefficient collaboration and integration of health information technologies (Health IT)
Gaps in communication among clinicians, patients and their families
A healthcare work system which, by design, does not adequately support the diagnostic process
To provide additional context, a review of 25 years of malpractice claims payouts in the U.S. by Johns Hopkins researchers showed that diagnostic error claims had a higher occurrence in outpatient (68.8 percent) vs. inpatient (31.2 percent) settings. However, those which occurred in an inpatient setting were roughly 11.5 percent more likely to be lethal. Total payouts over the 25 period amounted to a substantial sum of $38.8 billion.
To address these challenges many researchers and companies are leveraging artificial intelligence to improve medical diagnostics.
With the in vitro diagnostics market size projected to reach $76 billion by 2023, the aging population, prevalence of chronic diseases and the emergence of personalized medicine are contributing factors to the IVD market size. National health expenditures are estimated to have reached $3.4 trillion in 2016, and the health share of the GDP is projected to reach nearly 20 percent by 2025.
Current Applications of AI in Medical Diagnostics
Many of today’s machine learning diagnostic applications appear to fall under the following categories:
Chatbots: Companies are using AI-chatbots with speech recognition capability to identify patterns in patient symptoms to form a potential diagnosis, prevent disease and/or recommend an appropriate course of action.
Oncology: Researchers are using deep learning to train algorithms to recognize cancerous tissue at a level comparable to trained physicians. (Readers with a specific focus on cancer treatments may be interested in reading our full article on deep learning in oncology.)
Pathology: Pathology is the medical specialty that is concerned with the diagnosis of disease based on the laboratory analysis of bodily fluids such as blood and urine, as well as tissues. Machine vision and other machine learning technologies can enhance the efforts traditionally left only to pathologists with microscopes.
Rare Diseases: Facial recognition software is being combined with machine learning to help clinicians diagnose rare diseases. Patient photos are analyzed using facial analysis and deep learning to detect phenotypes that correlate with rare genetic diseases.
It is important to note that this is not a complete list of all diagnostic applications of AI. We aimed to present a succinct representative group of current initiatives based on our research.
We will now explore current applications of AI in medical diagnostics beginning with chatbots.
Chatbots
U.K.-based startup Babylon Health is a health subscription based service that has developed a chatbot for the prevention and diagnosis of disease.
Using speech recognition the chatbot will reportedly compare the symptoms that it receives from a user against a database of diseases. In response, it will recommend an appropriate course of action based on a combination of the reported symptoms, patient history and patient circumstances.
For example, the app’s response to someone describing flu-like symptoms might be a recommendation to visit the pharmacy for over-the-counter medication. In contrast, if more serious symptoms are reported by a user, the app may recommend dialing an emergency hotline or going directly to the hospital.
In addition to its diagnosis feature, the app is also designed to integrate patient data from wearable devices to monitor vitals such as heart rate and cholesterol level.
Babylon is currently backed by $85 million in Series A and B funding from 9 investors including a mix of investment firms and individual investors such as Google’s DeepMind Learning project founders, Demis Hassabis and Mustafa Suleyman.
Building on its 150,000 registered users who currently pay £7.99 ($11.40) per month to access Babylon’s flagship one-on-one doctor video consultations from a pool of 100 doctors (available 12 hours per day, 6 days a week) the chatbot is projected to run about £4.99 ($7.10) per month.
The chatbot has been offered for testing to an eligible pool of 21,500 patients across two hospitals. The startup has received registrations from 10 percent of these patients to test the app. Reportedly, both Essex hospitals have experienced a reduction in patient waiting times since Babylon was first made available for free to their patients back in April 2015.
However, it is unclear by how much the waiting times were reduced.
Described as a “personal health companion,” Berlin-based Ada Health offers a platform that uses AI and machine learning to track patient health and offer users a better understanding of changes to their health. The platform is offered to individual users, organizations and physicians.
While the startup does not describe itself as a diagnostic service per se, the app does provide recommendations based on patient symptoms and health information.
Aside from reviews published on the startup’s website and those posted by users on sites where the app is available for download, specific data on the impact of Ada’s platform on patient outcomes or an understanding of its funding model are not readily available.
In some ways, chatbots in the healthcare setting are a bit like IoT for the fitness space a few years ago. It seems inevitable, it seems to have great promise, it seems to make sense, but exactly what tools are used by what parties to get the results… we just can’t tell yet. In the coming 2-3 years I suspect we’ll see successful use-cases emerge.
Some of our past AI podcast guests have had guesses about what the healthcare chat interface experience might be like:
“Five years down the road I wouldn’t be surprised if before you see a doctor, you talk to a chatbot…and become an informed patient before.” – Riza Berkan, founder exClone Inc.
Oncology
Stanford University researchers have trained an algorithm to diagnose skin cancer using deep learning, specifically deep convolutional neural networks (CNNs). The algorithm was trained to detect skin cancer or melanoma using “130,000 images of skin lesions representing over 2,000 different diseases.”
In the U.S., there are approximately 5.4 million new skin cancer diagnoses each year and early detection is critical for a greater rate of survival. For example, early detection correlates with a 97 percent five-year survival rate but quickly decreases with later stages, hitting the 15-20 percent margin at stage IV. In 2017, an estimated 9,730 people will die of melanoma and one person dies of melanoma every 54 minutes.
To provide context, a visual examination is the first step of a skin cancer diagnosis and a dermatologist inspects a lesion of interest with the assistance of a dermatoscope (handheld microscope). If the dermatologist believes the lesion is indeed cancerous, or if the initial evaluation is inconclusive, the dermatologist will follow up with a biopsy.
Stanford’s deep learning algorithm was tested against 21 board-certified dermatologists who reviewed a reported 370 images and were asked if “they would proceed with biopsy or treatment, or reassure the patient” based on each image. Results showed that the algorithm had the same ability as the 21 dermatologists in determining the best course of action across all images.
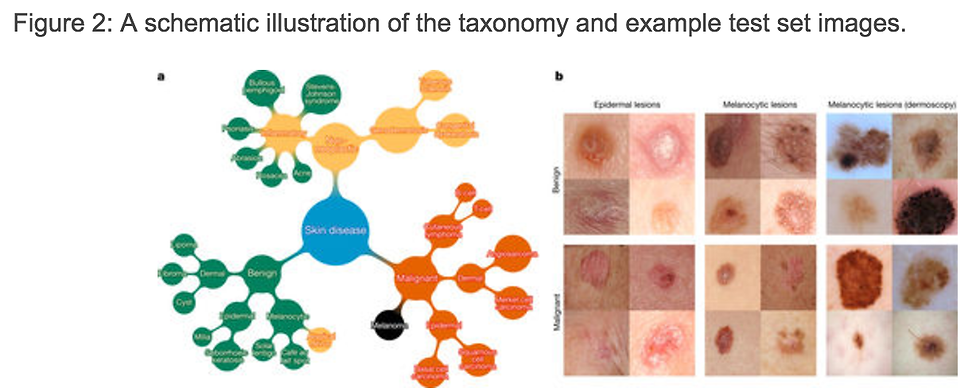
These are promising results, however the research team acknowledges that additional, rigorous testing is required before the algorithm can be integrated into clinical practice. Our research did not provide evidence of any clinical applications at this time.
More insights and a great number of use cases on AI programs in Oncology can be seen in our full length report on that one subject.
Pathology
The way pathologists diagnose diseases, which involves manual observation of images under a microscope, has remained relatively unchanged for over a century. In an effort to improve speed and accuracy of diagnoses, a team of researchers hailing from Beth Israel Deaconess Medical Centre and Harvard Medical School have used deep learning to train an algorithm capable of integrating multiple speech recognition and image recognition to diagnose tumors.
The researchers began with hundreds of images with labeled regions showing cancerous and noncancerous cells. The labeled regions were then extracted resulting in millions of examples that served as the basis for the model that would train the algorithm.
When compared to human pathologists, study results showed that the algorithm achieved a diagnostic success rate of 92 percent; four percentage points lower than the human rate of 96 percent. However, when the algorithm and human results are combined, an accuracy rate of 99.5 percent was achieved.
Our research did not provide evidence of any clinical applications, however these results may lead to wider adoption of deep learning methods in the field of pathology in the near future.
Rare Diseases
Through the Face2Gene app, facial recognition software is being combined with machine learning to help clinicians diagnose rare diseases (in this case, from facial dysmorphic features). Patient photos are analyzed using facial analysis and deep learning to detect phenotypes that correlate with rare genetic diseases.
The platform is currently available only to trained clinicians to prevent false positives and supports over 7,500 disorders. Face2Gene lists a number of partners on its website including Baylor College of Medicine, Cleveland Clinic and Kaiser Permanente. However, it is unclear how many total users are accessing Face2Gene and what impact the application has had on these patient populations.
The video below is a complete walk-through video of the Face2Gene software. At about 6:30 into the video, there is a brief demonstration matching a child’s face to various syndromes that the child may have based on facial analysis:
Concluding Thoughts on Diagnostic ML / AI
Machine vision is emerging as a common thread across these diagnostic applications, and it should be noted that improvements in that field will correlate closely with reliable applications in diagnostics. However, the process of trial and error will greatly influence the value of this technology in the real world and the degree to which it will be implemented in the field of diagnostics.
Applications of AI in medical diagnostics are in the early adoption phase across multiple specialties with limited data currently available on patient outcomes. These applications have the potential to impact how clinicians and health care systems approach diagnostics and the ability for individuals to understand changes to their health in real-time.
With projected rapid growth in the medical device sector, companies making efforts to bring accurate and reliable medical diagnostics based on machine and deep learning applications to market may be poised to capture a percentage of this profitable market (the huge venture investments the healthcare AI sector would seem to suggest that AI stands a chance to make a dent in the next wave of medical diagnostic tech).
While there is great promise, AI in medical diagnostics is still a relatively new approach, with many clinicians still left to be convinced of its reliability, sensitivity and how it will be practically integrated into clinical practice without undermining clinical expertise.
“The [healthcare teams] that are most exciting are the ones that include both health care professionals and AI professionals, because in general the AI people show up saying “as soon as someone gives us lots of data we’re going to find all the secrets”, and then the healthcare professionals don’t really know what’s possible — when you get them together you start to see some compelling applications…” – Steven Gullans
Continued rigorous testing of these applications will be necessary to validate their utility combined with education of clinicians and healthcare systems around how to effectively implement these technologies in clinical practice.
We will continue to follow the field of the medical diagnostics closely as we suspect this will be an active field for more AI applications in the near future. Stay tuned to our healthcare section for our latest healthcare coverage, research, and interviews.
Related Healthcare Interviews from the AI in Industry Podcast
At Emerj, we focus on presenting real-world AI applications and hard facts to our audience of business leaders. Our AI Opportunity Landscape research filters the “hype” and headlines to deliver insights on the real economic and strategic advantages of AI today and in the near future.
Through our “AI in Industry” podcast we present interviews with executives, investors and researchers, to equip our audience with knowledge of real trends and challenges of applying AI and their economic impacts.
The podcast is easily accessible on iTunes, and written summaries presenting interview highlights can be found in the “Interviews” section of Emerj.com. However, we have selected some interviews that may be of interest for readers who enjoyed this article on medical diagnostics:
Excel Venture Management’s Steve Gullans explores practical considerations in healthcare technology adoption, reasons that are often less about quality or potential of the technology and more about clarity on ROI for investors.
Catalia Health’s Cory Kidd shares predictions regarding five-year trends in medical AI applications.
exClone’s Dr. Riza Berkan discusses chatbots and touches on the future applications of what might manifest in this field in 5 to 10 years in medical and other consumer applications.
Yufeng Deng, Chief Scientist of Infervision, discusses expanding the capability of machine vision, including what kind of data one needs to collect and what is now possible with the technology.
Watch this:https://www.youtube.com/watch?v=Z5vxRC8dMv