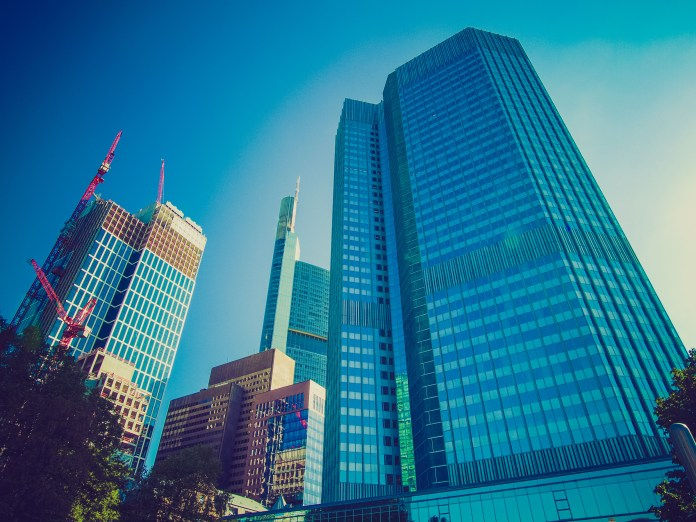
Verifying customer identity sounds straightforward, but despite the simplicity of the concept, Know Your Customer (KYC) compliance has become one of the most complex, consuming, confusing and costly processes for modern-day financial institutions.
In the US alone, anti-money laundering (AML) compliance costs for financial institutions are a sky-high $25.3 billion annually. Of course KYC is a subset of this, but despite significant spend and staffing investments, KYC workflows are falling short of their desired outcomes. Heard of Danske Bank’s ongoing money laundering crisis? Or ING Bank’s $900 million fine for AML reporting failures? What about the “Russian Laundromat”? Cases like these aren’t going away. Rather, they’re becoming more frequent as regulations expand and enforcements increase more quickly than banks can adapt.
Customer experience and business growth are also suffering at the hands of lengthy KYC processes. Analysts sort through enormous volumes of internal and external data to identify customers and monitor for risky activity in line with regulators’ expectations. That takes time, requiring up to a shocking three months to onboard a new client — and some applicants aren’t sticking around. In the UK, it is estimated that 25 percent of applications are abandoned due to KYC friction.
Statistics like these are staggering, and more than a bit overwhelming, but there is a way forward to greater efficiency and reduced risk within the financial services industry. Already, artificial intelligence (AI) and automation are becoming the go-to options for addressing the troubles of modern-day AML and KYC, and regulators are encouraging the adoption of innovative solutions. The biggest draw is AI’s ability to interpret, synthesize and correlate vast amounts of data, a game-changing contribution to the ongoing battle against financial crime.
Fashionable or functional: applying AI to KYC
By some counts, adopting regtech solutions for AML/KYC compliance could save banks an estimated £2.7 billion per year. Automation, process and IT are a big part of this and so is AI, but as with all technology solutions, AI is a means to an end, not a venture that makes sense on its own merit. When building an AI strategy, it’s important to identify expected outcomes and evaluate how AI can be applied to achieve specific goals. Here are the four pieces of lowest hanging fruit in AI for KYC that will bring the ROI.
1. Turning down the noise with natural language processing
Unstructured data is a critical source of information in the KYC investigation process. To date, most banks use traditional keyword syntax to identify relevant information from online publications, social media, public databases and more. This form of query, however, pulls all mentions of an organization or individual—whether relevant to the investigation or not—leaving analysts with far too much information to parse through.
Instead of simply pulling information, natural language processing (NLP), a form of artificial intelligence, systematically analyzes unstructured text to present analysts with only information that is risk relevant, eliminating the rest. NLP not only makes customer due diligence more efficient, it also makes it possible to build more robust risk profiles and enhance the discovery of ultimate beneficial ownership. The technology can digest huge volumes of data to identify sources that may have been missed in the past.
2. Clustering and consolidating research through advanced AI technologies
A standard KYC practice is conducting adverse media searches to pull up news coverage of an organization or individual that could influence their risk profile. Such a search brings back dozens of news articles from multiple publications all discussing the same event or topic.
Rather than requiring compliance teams to comb through each source to identify relevant information, AI solutions have the ability to interpret and condense data into a single story for analysts to review, introducing big time savings for compliance teams. AI tools consolidate relevant details to present the event, topic and entities involved in a digestible format, with the sources included for reference.
3. Reducing false positives with intelligent segmentation
Banks rely on rules-based KYC monitoring systems to detect suspicious transactions indicative of money laundering. Violation of a rule, such as an unusually large cash deposit, generates an alert for an analyst to review.
However, 95 percent of alerts are closed as false positives in the first phase of review, according to Reuters. A further 98 percent of alerts do not result in a suspicious activity report (SAR) or suspicious transaction reporting (STR). Manual review of millions of false positives is unsustainable, costly and takes away time analysts could instead spend on investigating higher-risk clients.
AI solutions will enable banks to evolve from a rules-based approach, widening the range of attributes used to rule hits in or out. Instead of using preset categories, AI algorithms will be taught to conduct intelligent segmentation, use machine learning to analyze transactions and identify suspicious behaviors. By learning from patterns, trends and previous matches, AI for AML will detect and recognize predictors of crime and assign risk ratings accordingly. A transaction that is normal for one client won’t be flagged, while the same type of transaction will raise an alert when anomalous to a different client’s risk profile.
Such monitoring and learning capabilities will help to significantly reduce the number of false positives, which remain a huge sticking point in both AML and KYC processes.
4. Automating the narrative through natural language generation
Ongoing monitoring of clients is an essential component of customer due diligence. As beneficial ownership structures change and clients move on and off PEP, sanctions lists and more, artificial intelligence and data automation will detect changes, natural language generation (NLG) will turn these data changes into actionable information and documented outcomes. Much like NLP in reverse, NLG software works like a human analyst, gathering relevant data and turning it into a story that is used to automatically update risk factors and write a narrative based on confirmed data.
Artificial intelligence is rapidly approaching to a place where it can materially address KYC challenges and set up banks for future growth. When applied strategically, it gives banks the ability to manage enormous volumes of data, keep up with regulations, identify sophisticated forms of financial crime, and attract new customers through a user-friendly onboarding experience.
Watch This : https://www.youtube.com/watch?v=Z5vxRC8dMvs