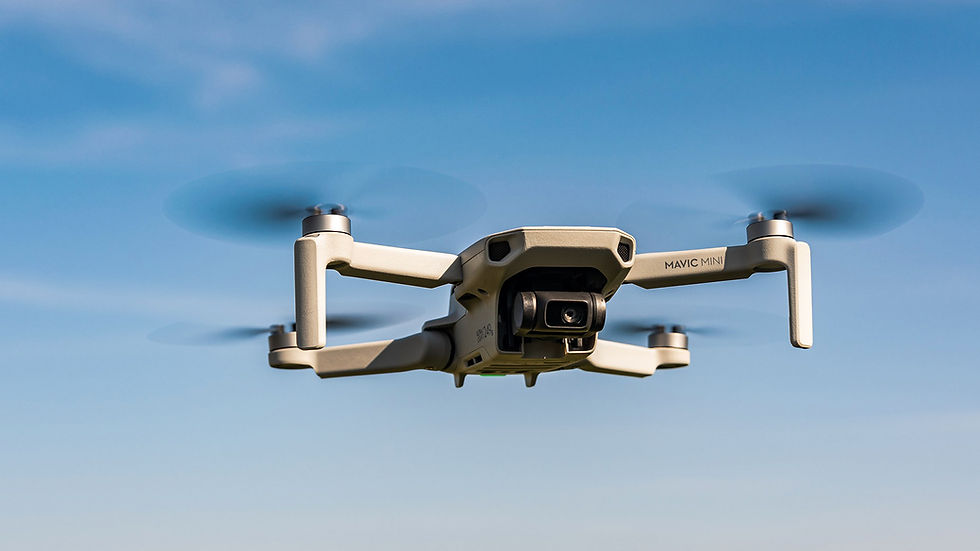
Digital transformation is no longer a fuzzy buzzword in industry, rather it is now a well understood and a credible approach to achieving business value. With increasing maturation of transformative technologies, it’s becoming a lot easier for organizations to chart their approach and digital transformation journeys.
The oil and gas industry was slow to leverage transformative technologies like the Internet of Things, machine learning, blockchain, artificial intelligence, and virtual reality. However, progressive companies have started to experiment with these new technologies to drive incremental value for their organization. These early adopters are showing how digital transformation is driving cost reduction, improving reliability, and increasing safety of people across the industry value chain and, in the process, attracting more companies and investment in these technologies.
Key challenges
The oil and gas industry faces the unique challenge of ensuring the efficient and safe operation of assets that are distributed geographically or in areas that are not easily accessible. In these cases, technologies like drones and machine learning could become very relevant. Drone-based aerial surveys of inaccessible areas can provide rich insights into the condition of the assets. Well platforms or areas above and near underground pipelines are some of the places where drone based inspection can work wonders.
How drones and machine learning can help overcome challenges
A common and simple use of a drone is to inspect inaccessible areas that would typically require scaffolding, rope, or a physical setups. By taking pictures of assets, such as flares, refinery columns, offshore platforms, or large crude oil tanks, and using them for visual inspections, oil and gas companies can prioritize detailed inspection and maintenance activities.
However, drone inspection’s true potential can be unleashed if machine learning is used to analyze the large volume of images to identify patterns and/or map the images to look for abnormalities. In this regard, a deep-learning algorithm based on a convolutional neural network (CNN) can help. In machine learning, a CNN (or ConvNet) is a class of deep, feed-forward artificial neural networks used for analyzing visual imagery. Simply put, it’s learning based on imagery.
In an oil and gas installation, a CNN-based algorithm running on a geo service-enabled machine learning platform can be used to create a digital representation of a remote platform, a crude oil tank farm, an over-ground layout for an underground pipeline, etc., by feeding standard images (a test data set) to train the algorithm to identify an asset on the ground. This enables a CNN algorithm to understand the details in imagery. Any new photograph captured with drone-based inspection can then be evaluated based on the CNN algorithm
For example, standard images of the surface over an underground pipeline can be fed into the algorithm to train it. Afterwards, every time a visual survey is done, the new images can be analyzed based on the learning in the CNN algorithm, and any abnormality that can’t be mapped with the existing data set can be highlighted in the analysis. Human intervention can target this exception for inspection, instead of reviewing the entire information.
Summary
Drone-based aerial imagery has the potential to significantly transform maintenance and inspection processes for oil and gas installations. A geo service-enabled machine learning platform with a CNN-based algorithm can analyze the results from aerial inspection, and recommend human intervention only if there are mismatches between the new imagery and the imagery used for training the algorithm.
B-AIM