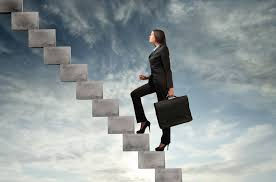
Every day, in banks across the world, dedicated risk experts investigate suspicious activity that is alerted within the financial system. Minute by minute, they are generating insights about customer behaviour, accruing risk knowledge and making risk judgements. Right now, most of this knowledge remains inside the heads of risk investigators, the ultimate black box. But what would be the potential for Financial Crime and Compliance (FCC) transformation if we could capture and put to use those insights from every expert risk investigator?
Aggregating customer data is driving transformation
Big data, Robotic Process Automation (RPA), Analytics and Machine Learning have driven an explosion of development in machine risk assessment within FCC. Significant progress has occurred in the last three years as banks have begun to overcome the initial inertia inherent in legal, political and technology issues.
In many cases, the entire technology stack of alert detection and risk scoring has been rebuilt. Rich sources of customer data have been consolidated and aggregated in easy to access data lakes. Multi-factor approaches to alert generation are now operational, using large scale bank data to model SARs probability, customer behavior anomalies and known scenario typologies to tune ever more accurate detection performance.
Many leaders in this space are already looking at the next source of rich FCC data – how the FCC compliance professionals in the organisation investigate and decision risk every day.
Applying investigator Decision Graphs at scale
New generation technologies that support the human risk analyst in conducting complex cognitive tasks are capturing how expert compliance analysts think at a granular level across tens of thousands of FCC investigations – also know as the ‘Decision Graph’ of an investigation.
For the first time, Bank Data Scientists, Risk Stewards and Operations executives have access to detailed Decision Graph’s for tens of thousands of alert investigations. Each one delineates the precise evidence trail, lines of enquiry and reasoning that led to different risk decisions with measures of probability and human expert consensus.
Figure 1: Example Decision Graph output from Nasdaq Automated Investigator for AML. The Decision Graph details the evidence that the AML Investigator has assembled and the relative influence of the evidence in recommending an ‘alert mitigated’ decision.
Banks are now beginning to answer entirely new questions to drive continued improvement in risk and efficiency. Some of the examples that we have come across include:
Identifying cases with similar patterns of investigation rationale where there is very strong expert consensus for ‘risk mitigated’ decisions
Identifying cases where expert judgement disagrees on key evidence and/or risk outcomes and analysing the detailed Decision Graphs to understand root causes
Exploring cases with similar patterns of investigation evidence where there is strong expert consensus for ‘non-mitigation’ and escalation for further investigation
Analysing specific areas of investigation evidence generation, such as categories of Transaction Purpose to reveal pathways to risk and efficiency
Measuring the actual decision boundaries where human experts judge different types of investigation evidence and the impacts on risk decisions
Shining a light on intractable problems
An often-cited challenge within FCC investigations is those instances where human experts will not agree on the same case rationale and risk decision. Automated investigation technologies are solving this problem by identifying many such cases where it is predicted that human expert consensus would be low. Through examining the detailed Decision Graphs for these cases, a bank can understand previously unidentified root causes and take proactive actions in advance of investigations.
For example, one bank showed higher levels of expert disagreement in cases where the source of funds was determined as non-salary employment income. Human experts varied significantly when classifying categories (such as dividends, directors’ loans, business expenses and rental income).
Moreover, even in cases when humans agreed on the classification of these different types of income, there was a noticeably higher variation on whether they would close the alert as non-suspicious or escalate for further investigation.
The bank was able to design initiatives in upstream risk management, operational controls and targeted human training to directly impact performance on these ‘contentious’ cases. This level of powerful insight into the cognitive decision making of the best risk experts allows investigation teams to become far more scientific and deliberate in the way they manage suspicious alerts.
New investigative insights driving FCC transformation
Detailed Decision Graph data provides Data Scientists, Risk Stewards and FCC Ops Execs with powerful new tools to improve risk management and operational efficiency through:
Tuning alert generation systems
Reworking operational systems
Validating hibernation systems and alerts
Targeted human training interventions
More precise risk management
Following the earlier waves of innovation from robotic processing and the aggregation of customer data, leveraging the power of human expert risk thinking at machine scale will be a key driver in the next wave of FCC transformation. It promises to be an exciting journey.
Watch This : https://www.youtube.com/watch?v=Z5vxRC8dMvs