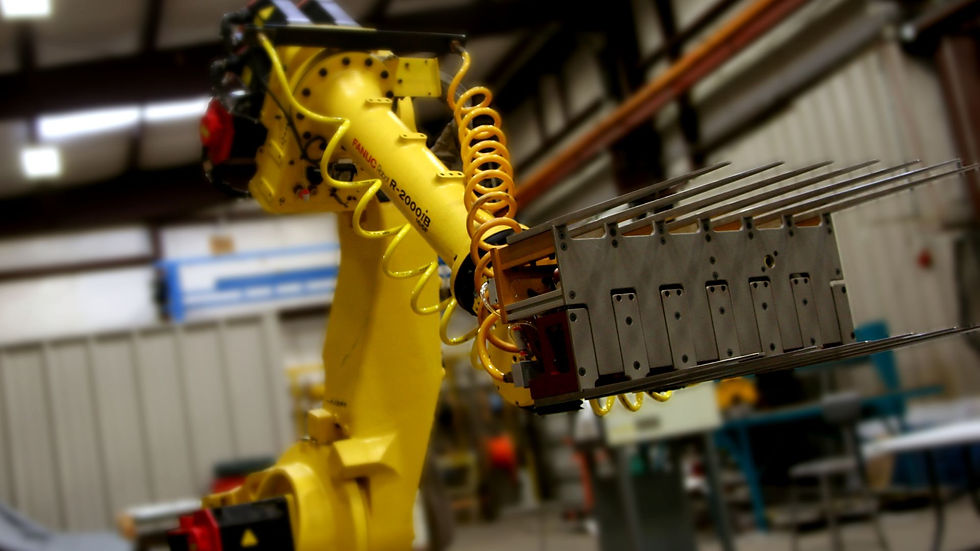
ndustrial Artificial Intelligence can hold the key to solving some of the most complex and painful manufacturing challenges. But every manufacturing process is unique – as are the challenges that come with them. And different types of manufacturing challenges require different types of AI to tackle them.
With all the noise in the market around “Industrial Artificial Intelligence”, “Industry 4.0”, “Smart Factories”, “Machine Learning” etc, it can be hard to figure out which AI technology is relevant to tackle your specific problems. In this mini-webinar — the first in our new Vlog series — we reveal a simple but effective methodology to help you make sense of it all.
Industrial Artificial Intelligence can hold the key to solving some of the most complex and painful manufacturing challenges. But every manufacturing process is unique – as are the challenges that come with them. And different types of manufacturing challenges require different types of AI to tackle them.
With all the noise in the market around “Industrial Artificial Intelligence”, “Industry 4.0”, “Smart Factories”, “Machine Learning” etc, it can be hard to figure out which AI technology is relevant to tackle your specific problems. In this mini-webinar — the first in our new Vlog series — we reveal a simple but effective methodology to help you make sense of it all.
Transcript:
[Eden] Hi everyone, my name is Eden. Welcome to the Seebo vBlog.
If you are in manufacturing, you’ve probably heard all the buzzwords: Industrial Artificial Intelligence, Smart Factories, Industry 4.0, Machine Learning. Today I would like to talk about a question we frequently hear from executives in manufacturing: How do you filter out the noise to get what you need? How can you find an Artificial Intelligence technology that actually addresses the business problem?
Today with me is Liran Akavia. As many of you probably know, Liran is the co-founder and the COO of Seebo. Liran is an expert on the field of industrial Artificial Intelligence and reducing manufacturing losses, and has extensive experience working with the world’s leading manufacturers.
Hi, Liran.
[Liran] Hello, Eden.
[Eden] So, Liran, how can manufacturers match their own unique production losses with the right artificial intelligence technology? Is there really a silver bullet out there or do they need to hunt for a specific solution for each individual problem?
[Liran] Manufacturers have diverse types of losses, and there is no silver bullet. There is no one artificial intelligence technology that you could deploy in your factory and will simply solve all the problems.
The good news is that there is a light methodology that every executive can deploy for their own teams to identify the relevant losses, and what is the relevant technology that works with that.
[Eden] Can you elaborate more about that methodology?
[Liran] Yeah, so… Just before we jump into the actual methodology I would like to share with you and with the audience how computers are thinking. Obviously we are not going to do a data-science lecture today, or not even a computer science lecture, but we are going to look in a very simplified way at the ways computers are looking at manufacturing problems, and once we understand that every manufacturing executive will be able to connect between the losses to the right technology.
So let me start with a quick couple of slides.
So let’s first take a look at how humans are solving manufacturing problems. So when we look at manufacturing problems we would differentiate between frequent problems and infrequent problems. When we have a frequent problem we have many examples of the problem and as humans we will try to look for a pattern, we ask what is common between all the problems and we will try to look for the common denominator in all those problems. In other cases, when we have infrequent problems, unfortunately we don’t have enough evidence for how the problem is looking, so we would ask completely different questions. Is it a normal behavior or an abnormal behavior?
Once we understand how humans solve problems, it is very easy to understand how computers are looking at problems.In the computer, or in the professional language,the frequent problems “world” will be supervised learning, and the infrequent problems “world” will be the unsupervised learning.
Obviously this is an over-simplification of the ways computers work. But generally, for computers, when there is a frequent problem, a computer would look at many examples of the problem and will try to look for a pattern, while when there are infrequent problems, the computer will ask a completely different question: Are we in a normal or abnormal situation?
[Eden] Okay, Liran, this is really helpful and very interesting, but how does this answer my question? How do manufacturers still find the right solution for their problem?
[Liran] You are right. Actually, let’s take a look at the methodology: So, the first step will be to list your production goals, all the losses you are looking to solve. It could be improving quality, or improving yield, or improving concentration, reducing downtime, increasing the asset reliability, process stability, energy consumption. Make sure that you have a very clear list and prioritize it.
With that list, take each one of the problems that you listed and put it somewhere around these quadrants.
So this magic quadrant goes on what drives the problem — process-driven or asset-driven — and what is the frequency of the problem: is it a high-frequency or a low-frequency problem?
Let’s take two classic examples. If I’m looking at bread baking, and on the weight of the bread. This is related to the process and usually high-frequency because the weight changes for every bread (loaf)/ The other extreme is a failing pump. It will probably fail once or twice a year, and the reason is usually the asset, is the pump itself. So the bread weight will be usually a process-driven, high-frequency problem, while the failure of the pump will be an asset-related, low-frequency problem.
So the first step was making a list of your problems. The second step, putting them on the quadrants, and the cool thing comes now. Each one of those quadrant areas is a different technology. Let’s start with quadrant one. In quadrant one we need artificial intelligence that understands the manufacturing process — after all, this is a process-driven problem — and we need supervised machine learning. If you remember what I said two minutes ago, the supervised machine learning is when we look at frequent problems and find the pattern, and explain why did it happen. On the other extreme, when we look at quadrant four, that was the pump example, it is an asset-driven, low-frequency problem. Here we usually use vibration or acoustic unsupervised learning, since we don’t have many examples, we simply ask if it is normal or abnormal and we do it per asset, not per process.
Those are usually the most important quadrants. Quadrant number one will be world of predictive quality and yield, quadrant number four will be the world of predictive maintenance.
Let’s take a look at quadrant number two. This quadrant does not have its own technology, but you can convert the problem to quadrant one or quadrant four. So, if you find a process proxy — I will explain what that is in a minute — go to quadrant one. If you don’t have a process proxy, go to quadrant four. Let’s take an example. Let’s take a vacuum system, this is an asset problem, that is failing every second week. It’s a frequent problem. So that would be quadrant two. If we can, for example, look for a process proxy that would be the pressure of the pump, and we know that the pressure of the pump is telling the story of the failing of the vacuum system, that would be a classic quadrant one example. But if we cannot find a process proxy like the pressure, we need to solve it with vibration or acoustic measurements.
As for quadrant (three), process-driven losses that are low-frequency, there is a technology behind that, but usually it is not a real concern for most manufacturers. An extreme situation in the process, very rarely, is not something that many manufacturers are actually concerned about. It’s not happening always and the technology of process-driven unsupervised learning is also not very accurate.
To make a long story short, generally your problems are probably in quadrant one or quadrant four. For quadrant one you will need predictive quality and yield, process-based technology which is supervised learning, and for quadrant four you will need predictive maintenance, vibration or acoustic unsupervised machine learning per specific asset.
Another thing that is super-important to know at this stage: no one can do it all. There is not one supplier that can tell you “I’m the best in the world in supervised learning and I’m the best in the world in unsupervised learning”. Messi is a great football player but he’s not the best in basketball. You can simply not… You cannot be the best in all.
[Eden] So essentially we would put the Seebo solution in quadrant number one?
[Liran] Yes, you are right. In Seebo, our entire world and expertise is in quadrant number one. Our algorithms are designed to solve problems that are process-driven and frequent. And because of the pure focus that we have and due to the fact that this is the only thing we do we are the best in the world at that.
[Eden] Thank you, Liran, and thank you everyone for all the great questions you’ve been e-mailing to us. Keep sending them in, the e-mail is right there.
B-AIM