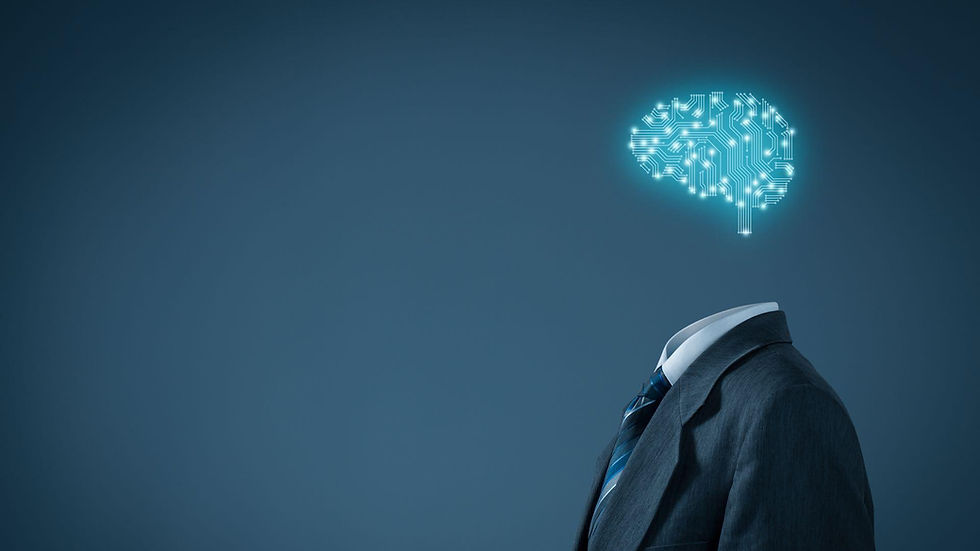
When you think about how to describe what a house is to a child, one approach might be to draw a four-sided square with a triangle on top and a door and a few windows and say, “that’s a house.” Another approach is to show that child various images of different houses and let the child begin to understand what a house is. Eventually that child might look at a similar building like an apartment complex for example, and innately understand the differences between the two.
By learning what a house is through images of houses, the child is learning by examples. This process is fundamentally at the heart of deep learning, especially for manufacturing.
Deep learning is a subset of artificial intelligence and a part of the broader family of machine learning. Instead of humans programming task-specific computer applications, deep learning takes unstructured data and trains itself to make more accurate outputs based on that training data. Deep learning applications learn and solve for narrow tasks without being explicitly programmed to do so.
Deep learning, then, isn’t just some far flung technology to help humans in the future. It’s solving problems, both mundane and important, right now: facial recognition to unlock phones or identify friends on social media photos, recommendation engines on streaming video and music services or when shopping at eCommerce sites, medical imaging such as helping diagnose diseases like cancer, spam filters in email, and credit card fraud detection.
Deep learning applications thrive in being able to consistently and at scale recognize anomalies and variance amongst a set of data. It’s something humans do intrinsically well, spot what’s different or understand what makes a house, a house, something that until now, computer systems based on rigid programming weren’t good at.
Humans, though, tire easily in their decision-making and computers do not. That’s why deep learning, if implemented for the right types of applications in conjunction with machine vision, can benefit factory and manufacturing companies in ways that other emerging technologies might take years to payoff.
Deep learning technology is being used to predict patterns and make critical business decisions. This same technology is now migrating into advanced manufacturing practices for quality inspection and other judgment-based uses such as defect detection or final assembly verification.
Much of deep learning is based on the work of neural networks. When a neural network is being trained, training data is fed to the bottom layer, the input layer, and it passes through succeeding computational layers, getting multiplied and added together in complex ways, until it finally arrives, radically transformed, at the output layer. This is where the program determines why one picture is a house and not, say, a dog.
The explosion of deep learning technologies owns itself in no small part to the explosion in popularity of modern video games. According to MIT, “the complex imagery and rapid pace of today’s video games require hardware that can keep up, and the result has been the graphics processing unit (GPU), which packs thousands of relatively simple processing cores on a single chip. It didn’t take long for researchers to realize that the architecture of a GPU is remarkably like that of a neural net.”
These modern, high-performance GPUs enabled the 50-layer neural networks of today, which has made deep learning applications possible. This gives manufacturing technology amazing new abilities to recognize images, distinguish trends, and make intelligent predictions and decisions.
B-AIM