With various amounts of healthcare data out in the field, Artificial Intelligence must efficiently sort through the presented data in order to “learn” and build a network. Within the realm of healthcare data there are two different types of data that can be sorted; unstructured and structured. Structured learning includes three different types of techniques including Machine Learning Techniques (ML), a Neural Network system, and Modern Deep Learning. Whereas, all unstructured data uses Natural Language Processing (NLP).
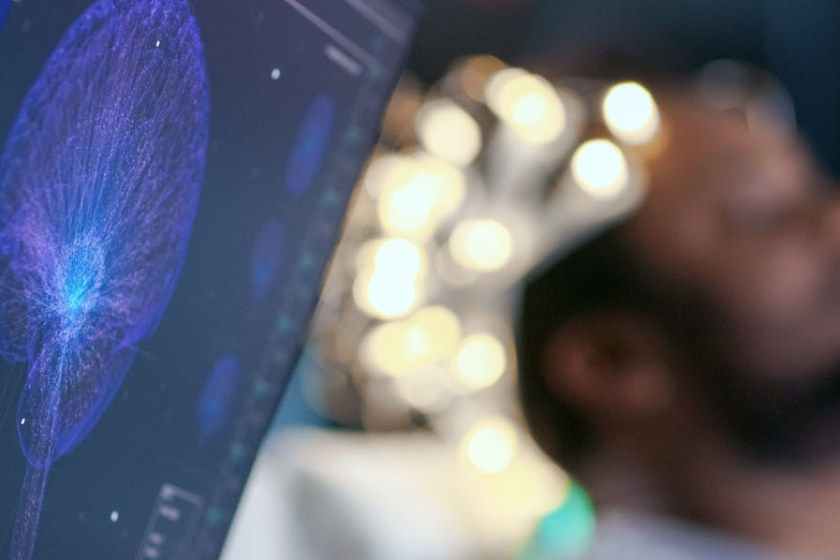
Machine Learning techniques use analytical algorithms in order to pull out specific patient traits, which include all the information that would be collected in a patient visit with a practitioner. These traits, such as physical exam results, medications, symptoms, basic metrics, disease specific data, diagnostic imaging, gene expressions, and different laboratory testing all contribute to the collected structured data.
Through machine learning, patient outcomes can then be determined. In one study, Neural Networking was utilized in a breast cancer diagnostic process sorting from 6,567 genes and paired with texture information inputed from the subjects’ mammograms. This combination of logged genetic and physical characteristics allowed for a more specific tumor indicator outcome.
The most common type of Machine Learning in a clinical setting is known as supervised learning. Supervised learning uses the physical traits of the patient, backed with a database of information (in this case breast cancer genes), to provide a more targeted outcome. Another type of learning used is Modern Deep Learning, which is considered to go beyond the surface of Machine Learning. Deep Learning takes the same inputs as Machine Learning, but feeds it into a computerized neural network; a hidden layer that further files the information to a more simplified output. This helps aid practitioners that may have multiple possible diagnoses narrow down to one or two outcomes; thus, allowing the practitioner to make a more definitive and concrete conclusion.
Similar to the structured data processes is Natural Language Processing, which focuses on all of the unstructured data in a clinical setting. This type of data is from clinical notes and documented speech to text processing when a practitioner sees a patient. This data includes, narratives from physical examinations, laboratory reports, and exam summaries. The Natural Language Processing uses historical databases that have disease relevant keywords aiding in the decision-making process for a diagnosis. Using these processes can provide a more accurate and efficient diagnosis for a patient, which in turn saves time for the practitioner, and more importantly can speed up the treatment process. The faster, more targeted and specific the diagnosis, the sooner a patient can be on the road to recovery.
watch this video - https://www.youtube.com/watch?v=-oDbVGficHw
Comentários